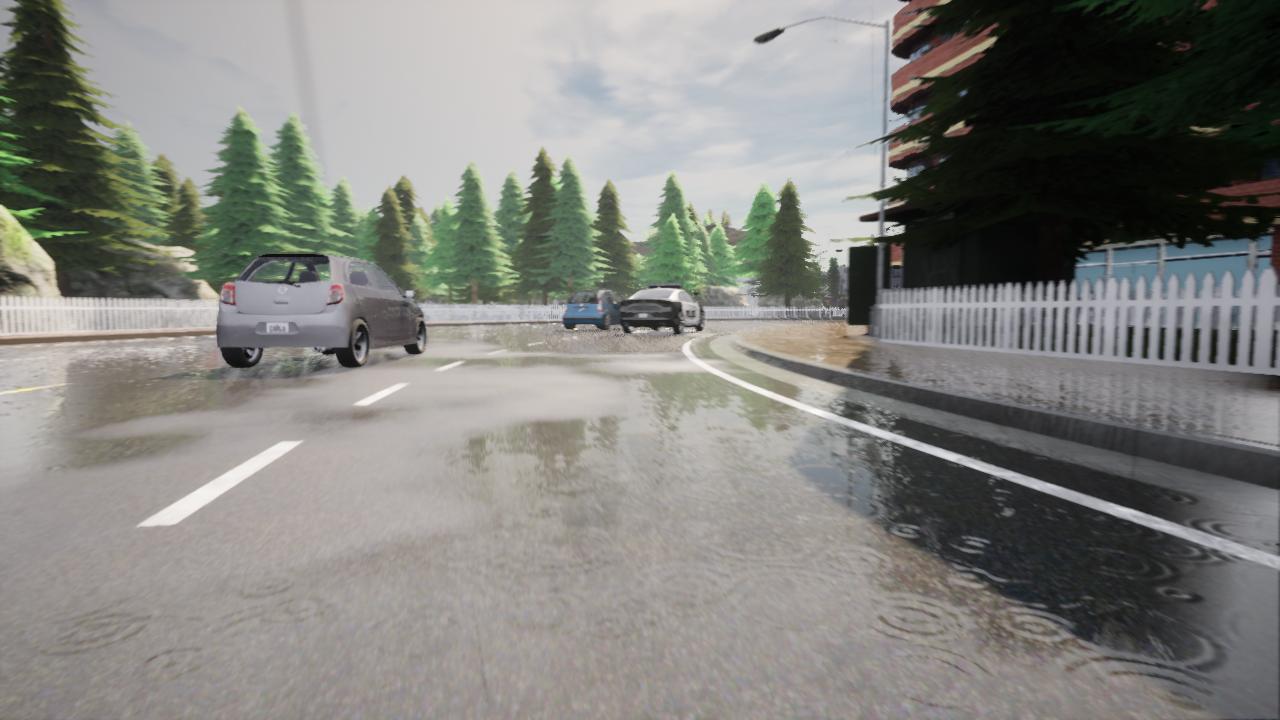
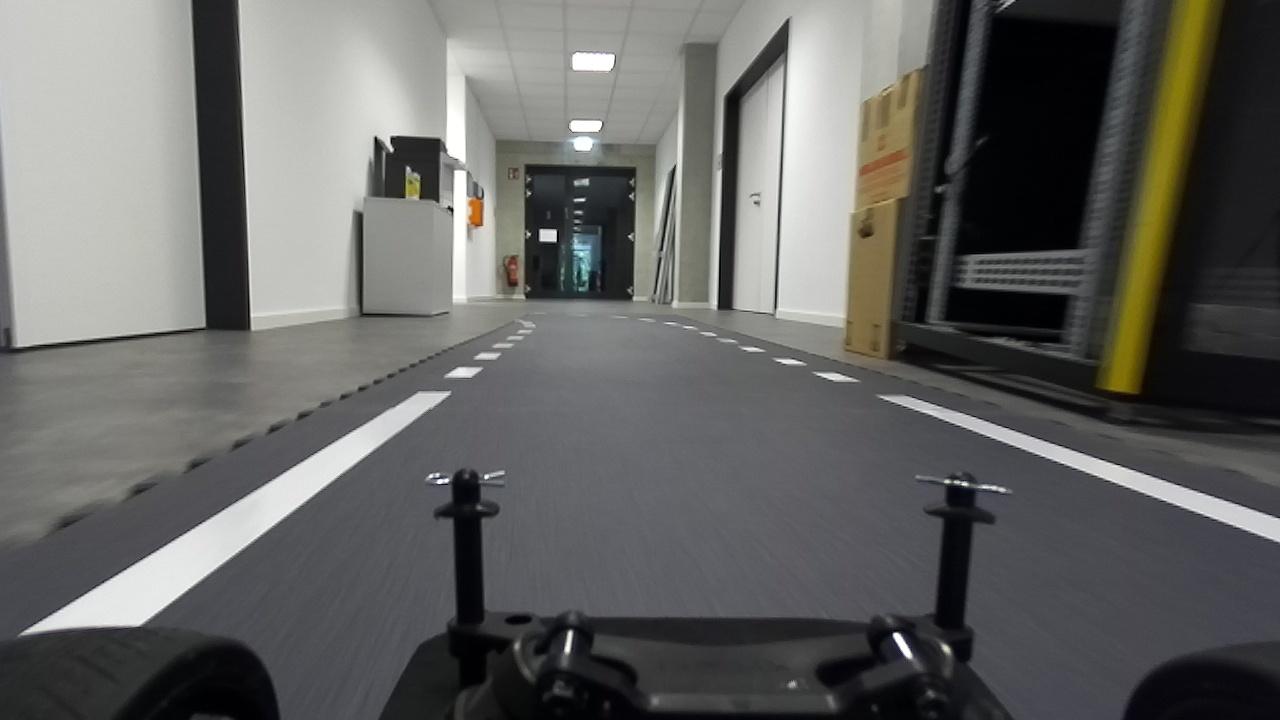
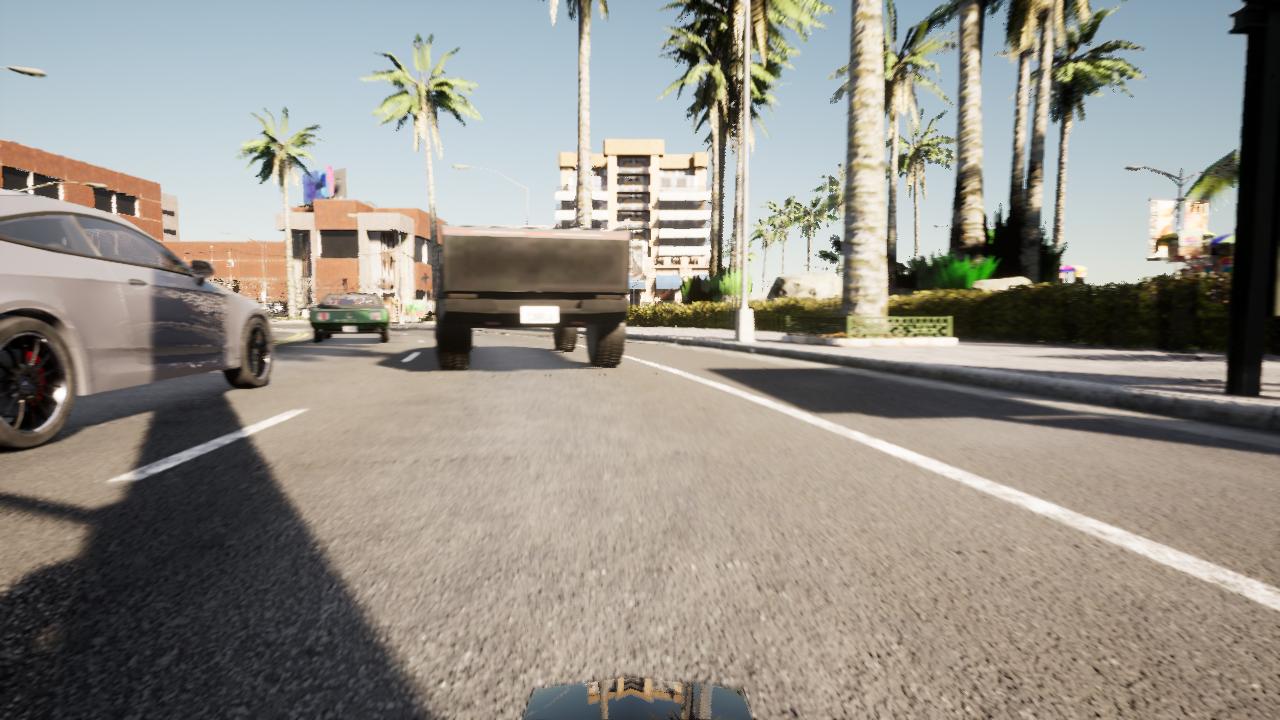
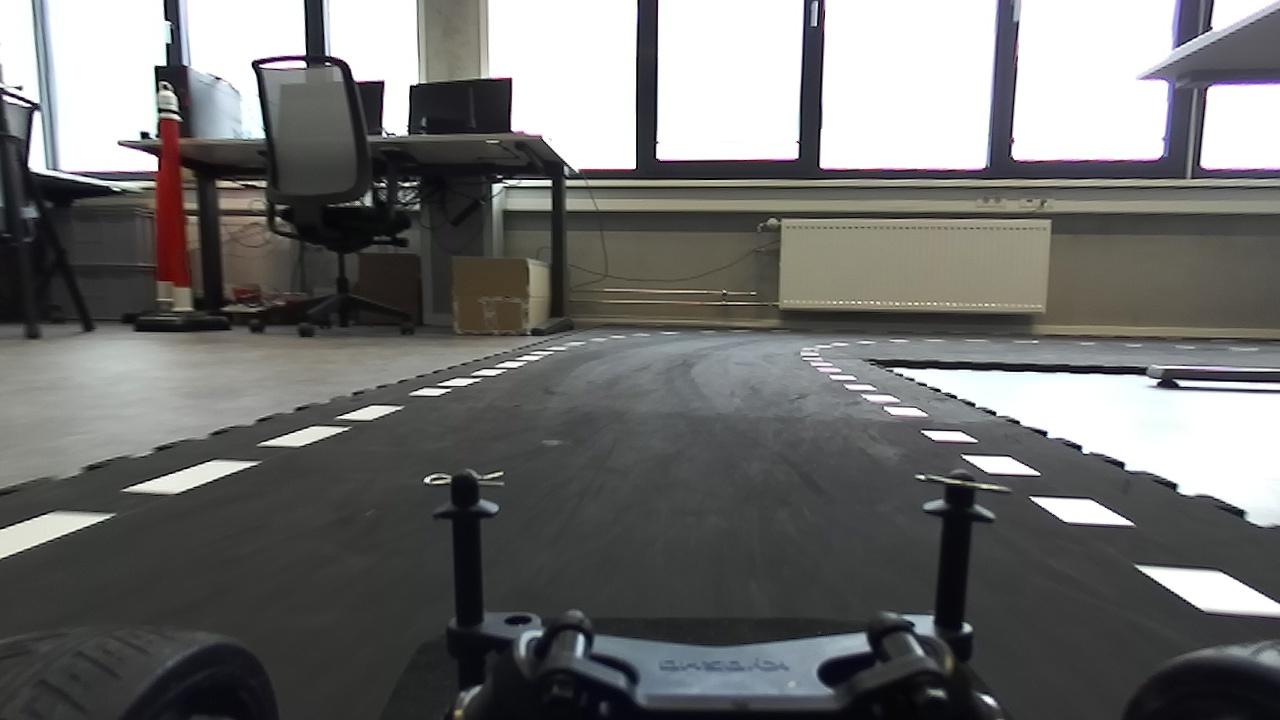
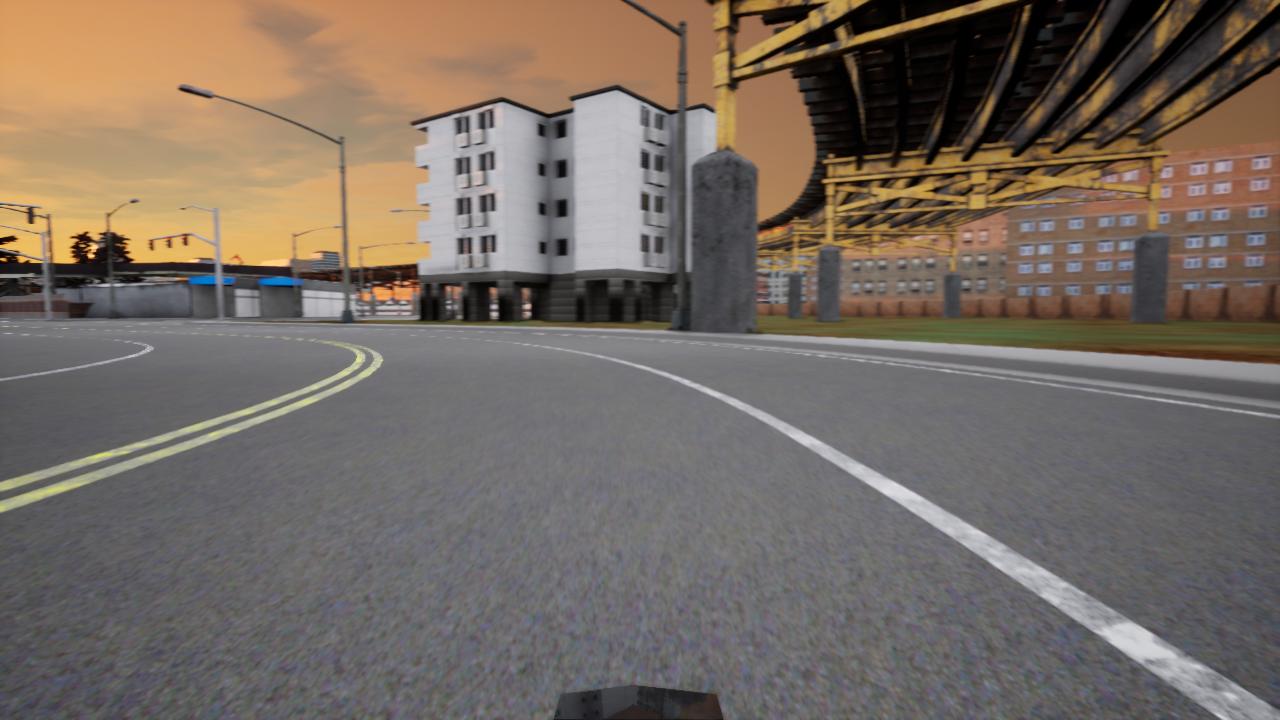
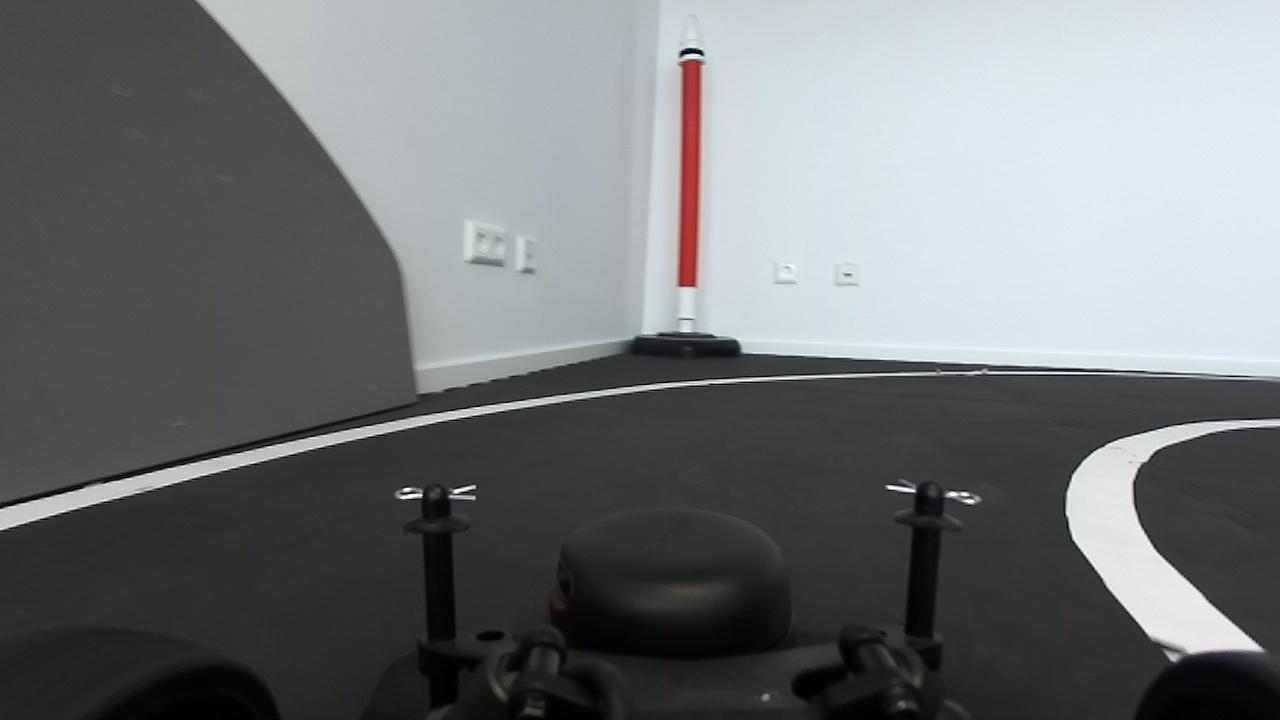
MoLane
Adaption from Simulation to Model Vehicle
Adaption from Simulation to Model Vehicle
Adaption from Simulation to Real Scenarios
Multi-Target Adaption from Simulation to Both
Source
Target
Source
Target
Source
Target 1 & 2
Dataset | Domains | Total Images | Train | Validation | Test | Lanes |
---|---|---|---|---|---|---|
MoLane |
CARLA Simulation Model Vehicle |
84,000 46,843 |
80,000 43,843* |
4,000 2,000 |
- 1,000 |
≤ 2 ≤ 2 |
TuLane |
CARLA Simulation TuSimple |
26,400 6,408 |
24,000 3,268 |
2,400 358 |
- 2,782 |
≤ 4 ≤ 4 |
MuLane |
CARLA Simulation Model Vehicle+TuSimple |
52,800 12,536 |
48,000 6,536** |
4,800 4,000 |
- 2,000 |
≤ 4 ≤ 4 |
*unlabeled **partially labeled
UFLD-SO
DANN
ADDA
SGADA
SGPCS
UFLD-TO
UFLD-SO
DANN
ADDA
SGADA
SGPCS
UFLD-TO
UFLD-SO
DANN
ADDA
SGADA
SGPCS
UFLD-TO
UFLD-SO
DANN
ADDA
SGADA
SGPCS
UFLD-TO
UFLD-SO
DANN
ADDA
SGADA
SGPCS
UFLD-TO
UFLD-SO
DANN
ADDA
SGADA
SGPCS
UFLD-TO
UFLD-SO
DANN
ADDA
SGADA
SGPCS
UFLD-TO
UFLD-SO
DANN
ADDA
SGADA
SGPCS
UFLD-TO
UFLD-SO
DANN
ADDA
SGADA
SGPCS
UFLD-TO
UFLD-SO
DANN
ADDA
SGADA
SGPCS
UFLD-TO
UFLD-SO
DANN
ADDA
SGADA
SGPCS
UFLD-TO
UFLD-SO
DANN
ADDA
SGADA
SGPCS
UFLD-TO
UFLD-SO
DANN
ADDA
SGADA
SGPCS
UFLD-SO
DANN
ADDA
SGADA
SGPCS
UFLD-SO
DANN
ADDA
SGADA
SGPCS
@inproceedings{NEURIPS2022_19a26064,
author = {Stuhr, Bonifaz and Haselberger, Johann and Gebele, Julian},
booktitle = {Advances in Neural Information Processing Systems},
editor = {S. Koyejo and S. Mohamed and A. Agarwal and D. Belgrave and K. Cho and A. Oh},
pages = {4046--4058},
publisher = {Curran Associates, Inc.},
title = {CARLANE: A Lane Detection Benchmark for Unsupervised Domain Adaptation from Simulation to multiple Real-World Domains},
volume = {35},
year = {2022}
}
10.34740/kaggle/dsv/3798459
CARLANE is licensed under the
Apache License 2.0
|
Apache License Version 2.0, January 2004 Copyright 2022 CARLANE Team (Julian Gebele, Bonifaz Stuhr, Johann Haselberger) Licensed under the Apache License, Version 2.0 (the "License"); you may not use this file except in compliance with the License. You may obtain a copy of the License at http://www.apache.org/licenses/LICENSE-2.0 Unless required by applicable law or agreed to in writing, software distributed under the License is distributed on an "AS IS" BASIS, WITHOUT WARRANTIES OR CONDITIONS OF ANY KIND, either express or implied. See the License for the specific language governing permissions and limitations under the License.